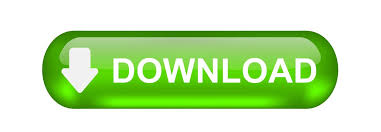
At this stage, airborne laser scanning has been most successfully adopted for forest inventory internationally, typically using area-based methods to characterize patches of the order of 0.04 ha in size ( White et al., 2013 Maltamo et al., 2014). Remote sensing offers the potential for high throughput, accurate, and spatially explicit phenotyping, providing an essential foundation for precision management ( Fahlgren et al., 2015). Such measurements are time consuming and error-prone.
#ASREML R BOUNDARY TRIAL#
Traditional forest inventory and trial measurement rely on ground-based measurement of traits such as tree diameter, height, and volume. This capability could not only be utilized in trials to support research into improved breeds for tree growth and quality, but also in assessment of trees for inventory of forest stands at different stages of the production cycle. A critical requirement for precision forestry is accurate and cost-effective methods to characterize individual trees ( Tsaftaris et al., 2016 D’odorico et al., 2020). The aspiration is to utilize improved tree breeds, planted on the most suitable sites, and managed to optimize production, while minimizing costs and environmental impacts by targeted applications of inputs such as fertilizers ( Dungey et al., 2018). The development of a precision approach to forestry can improve the efficiency and sustainability of managed forests. Individual tree phenotyping using an ACE approach is therefore recommended for analyses of research trials where traits are susceptible to spatial effects. The best ACE models provided statistically significant reductions in residuals ranging from −65.48% for tree height ( H) to −21.03% for wood stiffness ( A), and improvements in narrow sense heritabilities from 38.64% for H to 14.01% for A. Models including terms for spatial autocorrelation and competition performed the best and were labelled ACE (autocorrelation-competition-error) models. Models utilizing competition covariate terms were found to quantify previously unexplained phenotypic variation compared with standard models, substantially reducing residual variance and improving estimates of heritabilities for a set of operationally relevant traits. To examine our ability to account for environmental variation and its relative importance on individual-tree traits, we investigated the use of spatial models using ALS-derived competition metrics and conventional autoregressive spatial techniques. In this study we utilized airborne laser scanning (ALS) data to detect and characterize individual trees in order to generate tree-level phenotypes and tree-to-tree competition metrics. Phenotyping individual trees to quantify interactions among genotype, environment, and management practices is critical to the development of precision forestry and to maximize the opportunity of improved tree breeds.
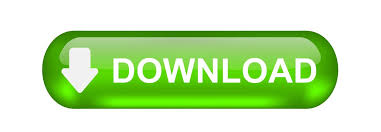